How to Tackle the Top Generative AI Challenges in Learning and Development by 2025
December 30, 2024
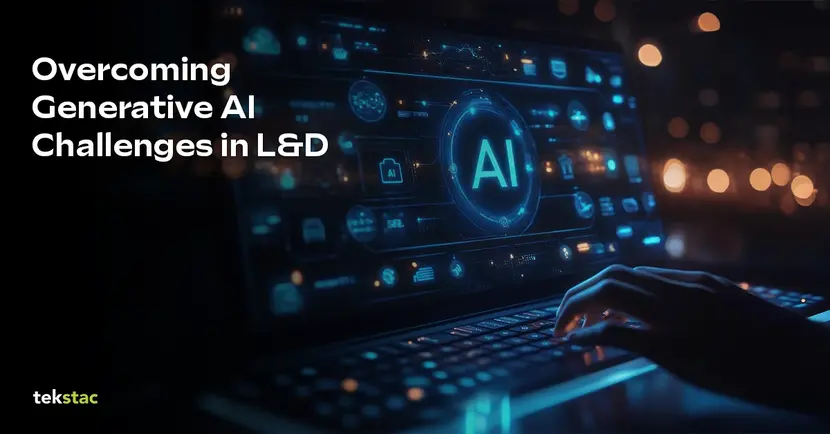
What if your training programs could truly adapt to every learner’s needs? Generative AI (Artificial Intelligence) is reshaping Learning and Development (L&D) by providing adaptive, scalable, and data-driven solutions tailored to diverse learner profiles.
Say goodbye to outdated, one-size-fits-all training. AI brings new possibilities, from creating personalized learning paths to delivering interactive content and automating assessments.
But as exciting as these advancements are, they come with challenges that demand attention. Tackling Generative AI challenges in L&D is essential for ethical and effective adoption by 2025.
Data Bias and Quality: Ensuring Fairness in Learning Outcomes
The first major challenge lies in tackling algorithmic bias and data quality issues. A 2024 report highlighted that 42% of organizations struggle with poor data quality, which undermines the accuracy of AI outputs.
Bias in datasets can perpetuate stereotypes and negatively impact the inclusivity of training materials. For example, biased AI models might produce content that unintentionally alienates certain groups, eroding trust and engagement among learners.
To overcome this, organizations must prioritize:
- Using diverse and representative datasets.
- Implementing proactive bias detection and correction measures.
- Conducting regular audits of AI systems.
These actions ensure fairness while maintaining the reliability of AI-driven learning systems.
Ethical Frameworks: Balancing Innovation with Integrity
One of the biggest Generative AI challenges lies in the transparency of generative AI models and algorithms. Often referred to as “black boxes,” they make it hard to understand how decisions are made, raising significant ethical concerns. This opacity can create dilemmas when it comes to accountability and trust. To address these issues, global powers like the U.S. and China are stepping up by advancing AI regulations aimed at promoting transparency and fairness.
For businesses, this means aligning with emerging ethical standards to ensure they not only stay compliant but also foster trust among their teams and stakeholders. Tackling these Generative AI Challenges with a proactive approach is essential for creating AI systems that are both reliable and equitable.
Key strategies include:
- Deploying explainable AI tools to foster transparency.
- Establishing real-time audits to monitor system outputs.
- Crafting policies that ensure fairness and inclusivity.
By embedding ethics into their L&D strategies, organizations can create AI-powered solutions that are both innovative and trustworthy.
Infrastructure and Integration: Bridging Legacy Systems with Modern AI
Integrating generative AI into existing systems presents technical challenges, especially for organizations reliant on legacy learning management systems (LMS). These traditional platforms often lack the flexibility required for dynamic AI tools, leading to compatibility issues and inefficiencies.
To address this:
- Adopt middleware solutions to bridge gaps between old and new systems.
- Transition to modern AI-first platforms that are built to overcome the shortfalls in traditional skilling tools.
- Collaborate across IT and L&D teams to align technical and educational priorities.
Such strategies minimize disruptions while enabling seamless integration of cutting-edge tools.
High Costs of Development and Deployment: A Path to High ROI
While the financial investment in generative AI can seem significant—ranging from $100,000 to $600,000 per project, according to a Scaleup Ally report—the potential return on investment (ROI) makes it a game-changer for Learning and Development.
AI-based training systems can streamline workflows, enhance learner engagement, and provide data-driven insights that improve program effectiveness, ultimately saving both time and resources.
To maximize ROI, organizations can:
- Focus on essential features through a Minimum Viable Product (MVP) approach.
- Utilize pre-built AI platforms and cloud-based services to minimize upfront expenses.
- Partner with technology experts for scalable, cost-efficient solutions.
By aligning investments with measurable outcomes, businesses can ensure that every dollar spent contributes to creating impactful and sustainable L&D programs.
Resistance to Change: Building Trust and Confidence
Integrating AI into Learning & Development (L&D) presents notable Generative AI Challenges, particularly concerning organizational resistance to change. Employees often view AI as a potential threat to their job security, while leadership may hesitate due to financial implications. A 2024 PwC report reveals that 39% of workers are apprehensive that AI could render their current skills obsolete.
To overcome resistance, organizations should:
- Promote open communication to alleviate fears and build trust.
- Invest in upskilling programs that empower employees to engage with AI confidently.
- Implement pilot programs to demonstrate the tangible benefits of AI.
Through collaboration and education, businesses can foster a culture of acceptance and enthusiasm for technological innovation.
Content Authenticity: Ensuring Accuracy and Credibility
Generative AI models occasionally produce misleading or plagiarized content, raising concerns about authenticity. A 2023 study introduced the Hallucination Evaluation benchmark for Large Language Models (HaluEval), revealing that models like ChatGPT generate hallucinated content—plausible yet unverifiable information—in approximately 19.5% of their responses.
Solutions include:
- Incorporating human oversight to review and validate AI-generated materials.
- Leveraging explainable AI to trace content origins and ensure transparency.
- Using AI watermarking technologies to verify authenticity.
By implementing these measures, organizations can maintain the integrity of their training programs.
Overcoming Algorithmic Bias
One of the most significant Generative AI Challenges lies in addressing algorithmic bias, which can undermine equitable learning opportunities and reinforce existing inequalities. For example, career guidance tools powered by AI might unintentionally push learners away from certain professions if the underlying data reflects societal biases.
Addressing this requires:
- Regular audits to detect and rectify biases.
- Engaging diverse voices during the design phase.
- Developing equitable algorithms that prioritize fairness and inclusivity.
These efforts ensure AI tools empower all learners, regardless of background.
Technological Evolution: Keeping Pace with Rapid Advancements
As AI technologies evolve rapidly, staying updated becomes a challenge for L&D teams. Traditional training models risk obsolescence if they fail to adapt.
Recommended strategies include:
- Investing in continuous upskilling for L&D professionals.
- Building flexible systems that accommodate new technologies seamlessly.
- Prioritizing inclusive design to address diverse learner needs.
By embracing agility, organizations can future-proof their L&D initiatives.
Lack of Personalization: Bridging the Gap for Individual Learners
Despite its potential, generative AI often struggles to deliver true learner personalization. Many systems rely on standardized datasets, which fail to account for individual preferences, learning styles, or career goals.
To address this, organizations should:
- Invest in adaptive learning platforms that tailor content to individual progress and performance.
- Use advanced analytics to refine training programs based on real-time feedback.
- Incorporate human oversight to balance automation with empathy.
These approaches create more engaging, relevant, and impactful learning experiences.
Language and Cultural Barriers: Enhancing Global Accessibility
Generative AI’s limitations in handling diverse languages and cultural contexts present significant challenges for multinational organizations. A 2024 report from the World Economic Forum highlighted that most AI chatbots and language models are trained on only about 100 of the world’s 7,000 languages, with English being the dominant focus.
Organizations can overcome these barriers by:
- Localizing training materials to reflect regional languages and cultural nuances.
- Training AI models on diverse datasets to improve multilingual capabilities.
- Involving local experts to ensure cultural sensitivity and relevance.
By addressing these challenges, businesses can create inclusive L&D programs that resonate with a global workforce.
Opportunities: Unlocking the Positive Impact of Generative AI in L&D
While it’s important to address the challenges, generative AI also opens up exciting opportunities in Learning and Development. By leveraging its capabilities, organizations can:
- Create Personalized Learning Experiences: AI tailors training to individual learners’ needs, boosting engagement and knowledge retention.
- Enhance Productivity with Automation: Automating tasks like content creation, assessments, and progress tracking saves time for both learners and instructors.
- Enable Real-Time Insights: AI-powered analytics provide actionable data, helping organizations refine their programs for better outcomes.
- Expand Global Accessibility: Multilingual and culturally adaptive AI tools allow organizations to reach a diverse global workforce effectively.
Conclusion
By solving the challenges of generative AI, organizations can create better Learning and Development programs that are fair, effective, and help everyone succeed.
For forward-thinking solutions inspired by industry-leading practices, explore a 360 skilling platform that innovative approaches to unlock the full potential of AI-driven L&D.